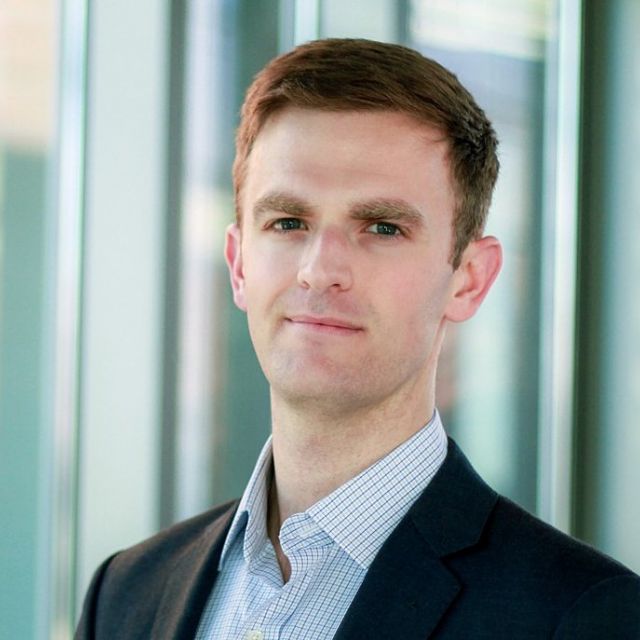
Aaron Meyer
Mechanistic and data-driven dissection of cell communication through tensor methods
Effective engineering requires the ability to group systems into modular components. When modularity can be identified, it becomes tractable to measure, model, and manipulate individual parts to control the overall system. Cell responses, unlike constructed systems, lack clear modularity—even “simple” pathways, such as an individual receptor-ligand complex, can display differing effects based on timescale, cell type, stimulation, the type of response measured, and context. Engineering cell systems for desired outcomes is therefore almost always constrained by an incomplete view of the overall pathway.
Dimensionality reduction methods can be extremely effective at identifying modular components of a system when this structure is not known a priori. These methods work to find a smaller approximation of a dataset by identifying patterns. However, these methods universally struggle with measurements collected across different modes, such as over time, cell types, and perturbations. While techniques such as U-MAP may reduce the data into patterns, they can remain a confusing mixture of these mode effects.
Tensor generalizations of dimensionality reduction provide solutions to this problem. Using several recent applications of these techniques across three length and complexity scales, I will show how these methods improve our ability to identify the mechanisms of and control cell response. In designing cell type-selective IL-2 therapies for autoimmune disease, analyzing serology responses to SARS-CoV-2 and HIV, or integrating data of infection response across ‘omic technologies, tensor methods help provide insight into the underlying biology. Specifically, tensor methods improve the extent to which we can simplify the data, separate mode (e.g., time, perturbation) effects, and integrate patterns across datasets. Finally, I will discuss some of the reasons that tensor-based methods remain limited in their bioengineering application. Resolving these limitations, and applying these methods in a more widespread manner, will help improve our ability to control cellular responses.
Bio: Dr. Aaron Meyer is an Assistant Professor of Bioengineering at the University of California, Los Angeles. After receiving his B.S. in Bioengineering from UCLA, Dr. Meyer completed his Ph.D. studies in Biological Engineering at the Massachusetts Institute of Technology and then went on to be an independent fellow at the Koch Institute for Integrative Cancer Research. Dr. Meyer’s research aims to develop new computational methods, deeply integrated with experiments, to understand intercellular communication and how it can be therapeutically manipulated. His lab focuses on combinations of mechanistic and data-driven modeling as a solution to our incomplete knowledge of cellular pathways, with applications in cancer and immunity where models can have immediate therapeutic impact. His work has been recognized by the NIH Director’s Early Independence Award, a Siebel Scholars award, a Hellman Fellowship, the UCLA Faculty Career Development Award, the Northrop Grumman Excellence in Teaching Award, and he has been named as Ten to Watch by the Amgen Foundation.